
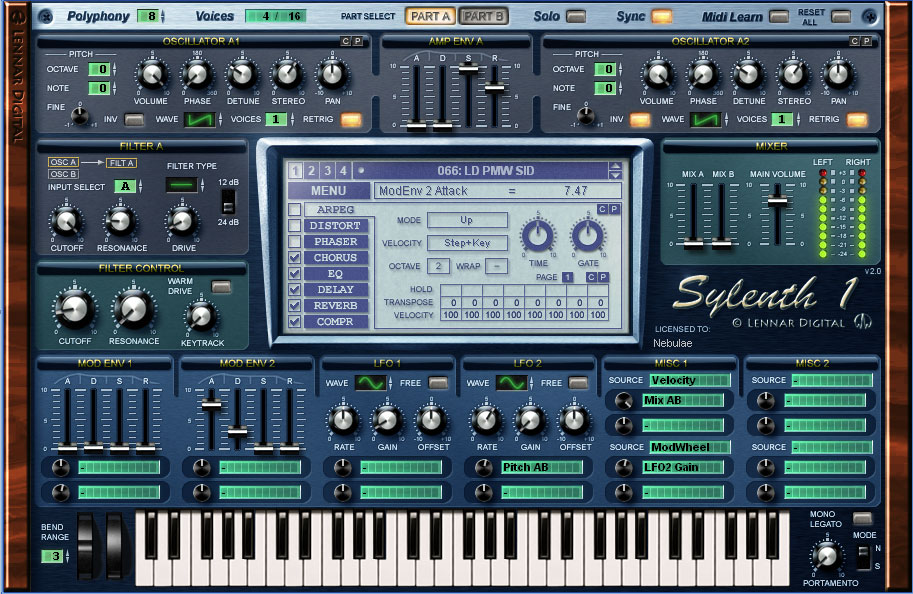
In an effort to be able to identify those patients with less favourable outcomes, we have recently discovered a novel radiomic signature of tumour phenotype and prognosis, derived from the segmentation of conventional preoperative CT imaging. It is known that despite obtaining total macroscopic tumour clearance at maximal effort upfront debulking surgery, ~25% of patients will relapse early, already in the first postoperative year. Radiomic algorithms based on high-dimensional quantitative features extracted from conventional imaging modalities have been demonstrated to represent a non-invasive platform to quantify tumour heterogeneity and determine prognostic signatures for operability and relapse. The concept of radiomics analysis is a promising novel approach in that direction. Still, reliable prognostic and predictive biomarkers that would allow the development of more personalised treatment pathways for patients are lacking.
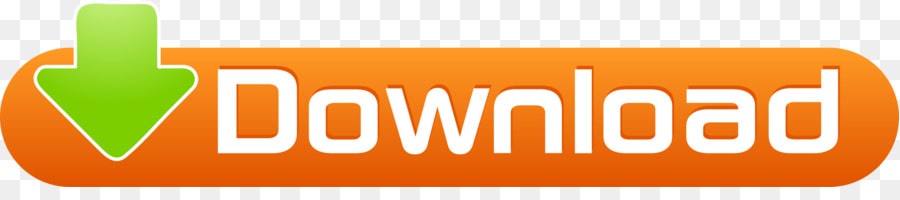
Through refinement of cytoreductive techniques, the introduction of novel targeted agents and the implementation of genetic and tumour profiling studies, our therapeutic strategies have evolved towards a maximal effort approach across multiple levels that has led to a significant improvement in patient survival. Surgical and systemic treatment of high-grade serous epithelial ovarian cancer (HGSOC) has experienced unprecedented advances over the last two decades. Further prospective, multicentre trials with a translational aspect are warranted for the incorporation of this radiomics approach into clinical routine. RPV was validated to independently identify those HGSOC patients who will not be operated tumour-free in an optimal setting, and those who will relapse early despite complete tumour clearance upfront. Moreover, lower RPV was significantly associated with total macroscopic tumour clearance (OR = 2.02 95% CI:1.56–2.62 P = 0.00647). High RPV scores independently associated with significantly worse progression-free survival (PFS) (HR = 1.69 95% CI:1.06–2.71 P = 0.038), even after adjusting for stage, age, performance status and residual disease. The distribution of low, medium and high RPV scores was 54.2% ( n = 175), 33.4% ( n = 108) and 12.4% ( n = 40) across the cohort, respectively. RPV was correlated with operability, survival and adjusted for well-established prognostic factors (age, postoperative residual disease, stage), and compared to previous validation models. RPV was calculated using standardised algorithms following segmentation of routine preoperative imaging of patients ( n = 323) who underwent upfront debulking surgery (01/2011-07/2018). Here, we wish to assess the prognostic value of the newly discovered Radiomic Prognostic Vector (RPV) in an independent cohort of high-grade serous ovarian cancer (HGSOC) patients, treated within a Centre of Excellence, thus avoiding any bias in treatment quality. Predictive models based on radiomics features are novel, highly promising approaches for gynaecological oncology.
